This is the last of a two-part series on the power of using Artificial Intelligence to optimize a profitable return on collections.
In PART I, we reviewed the RDS Asset Prediction Model which can be used to accelerate suit-decisioning by more accurately predicting a consumer’s assets. In today’s post we illustrate how the prediction model works in an actual suit-decisioning process.
CASE STUDY: APPLICATION OF SUIT-DECISIONING IN THE BANKING INDUSTRY To verify the performance of our PI suit-decisioning methodology, a top 10 bank in the US selected a test pool of accounts that had already been collected upon. The requested data points included account information with charge-off date, balance, age, consumer name, and address. The bank did not provide any information regarding collection figures for the accounts. The test pool included 1,495 accounts of mortgage, unsecured, and auto deficiency account types. The charged-off dates of accounts ranged from June 2018 to February 2019. The balance distribution of accounts with some statistics are presented in Figure 2.
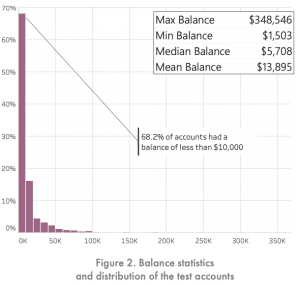
Given the requested information from the bank and our proprietary data pipelines, we gathered input variables to run our PI analysis. The client’s goal was to have a quick and cost-effective analysis on their accounts, hence, Eq. (3), predicted asset, was applied as the basis for PI suit-decisioning. The PI score for each test account was calculated and then summarized into a range of 10 deciles. These deciles were 10 groups, each consisting of the same number of accounts, ranked by their PI values. As an example, the first decile represented the 10% of accounts with the highest PI values, the second decile represented the next 10% with the next highest values, etc. The PI scores and deciles were sent to the bank so that they could evaluate the performance of our suit-decisioning methodology. The bank compared the account-level PI rankings to their actual account collection data. The bank then provided the collection data back to RDS to compile these results. A summary of the collection on the accounts, using their own collection strategy, is provided in Table 1. The total value of the pool was $20,773,770. Overall, 22.7% of accounts made payments totaling $2,564,068, which represented 12.3% of the total value.
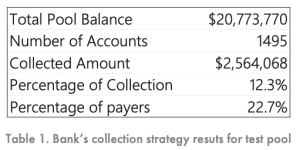
Debt Collection AI Case Study: Application of Suit-Descioning in the Banking Industry
The next step was to determine how PI would have helped the bank to prioritize collections efforts on this pool of accounts. Table 2 shows the accounts sorted by their PI deciles and compares that to actual collection amounts provided by the bank.
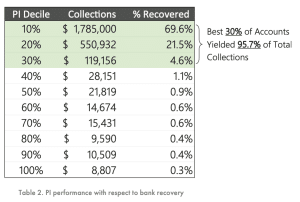
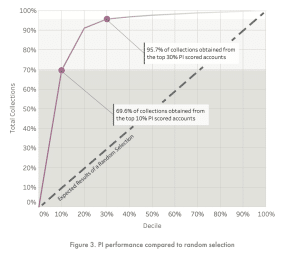
Table 2 shows the results, that higher PI deciles (i.e. first 3 deciles – top 30%) have resulted in significantly higher collections vs. the accounts in lower deciles (i.e. bottom 70%). To be specific, the top decile (top 10%) alone accounts for nearly 70% of the bank’s total collections. Figure 3 shows PI performance on this pool as compared to a random selection (where it would be expected that 30% of the accounts would yield 30% of the total collections). This means that if the bank had used PI, they could have targeted the top 30% of the accounts (i.e. top 449 accounts with highest PI values out of 1495 accounts) and yielded 96% of their total collections. The 70% of accounts with lower PI scores yielded a mere 4% of their total collections.
Clearly this performance is exceptional, and while we expected PI to outperform a random selection, this far exceeded the anticipated results. The performance of PI on in-house accounts is presented in Figure 4. From this figure, PI is expected to outperform random sampling that eventually verifies the significance of PI in cost effective suit decisioning optimization. However, the outperformance of PI over the bank’s accounts is still exceptionally significance.
CONCLUSION
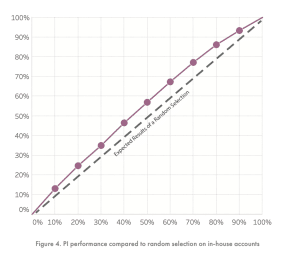
This two-part series introduced an AI based suit-decisioning methodology named PI. The proposed PI allows banks and other financial institutions to optimize their debt collection strategies by prioritizing their litigation decisions. PI was developed based on multi-task learning regime using multimodal dataset. The proposed methodology is equipped with an asset predictive modeling that allows for quick and cost-effective analysis. The effectiveness of the proposed methodology was verified based on analysis of a test pool adopted from one of the top 10 banks in the US. The results indicated that PI would significantly improve the bank litigation outcomes. Targeting only 30% of the accounts with the highest PI values, they would be able to recover 96% of their original collections. Although we do not believe that results such as these are typical, the results do confirm that PI is a powerful tool that financial institutions can use to prioritize their accounts in their collection strategies, maximizing collections and lowering costs.
To learn more about Recovery Decision Science, contact:
Kacey Rask : Vice-President, Portfolio Servicing
[email protected] / 513.489.8877, ext. 261